

Therefore, finding an appropriate method for signal denoising is an extremely important processing step before analysis and diagnosis. The inherent noise of the system and the structure of the microfluidic chip will cause the ME-C 4D signal to be disturbed, thus affecting the analysis of the detection results and reducing its accuracy. But the ME-C 4D device has poor anti-electromagnetic interference ability and low sensitivity. Therefore, it can effectively avoid some troubles of electrochemical contact detect method, such as electrode scaling, electrolysis bubble, electric field interference, and so on. The ME-C 4D device analyzes the ion composition in solution by detecting the change of electrical conductivity based on the coupling capacitance between the electrode and insulation layer of the chip. Because of its advantages of little samples and reagents consumption, fast analysis speed, high separation efficiency, and convenient miniaturization, microfluidic technology has been widely used in different fields, such as biomedicine, food inspection, environmental monitoring, clinical application, and so on. Microfluidic technology, especially microchip electrophoresis based on capacitively coupled contactless conductivity detection (ME-C 4D), has become a very important and promising branch of miniaturized total chemical analysis systems ( μ-TAS).

After executing this method, the noise is cleanly removed, and the signal peak shape and peak area are well maintained. This method has been successfully applied to the self-developed ME-C 4D equipment. Furthermore, the denoising effect is compared with, as well as proved to be superior to, the existing techniques, such as Savitzky–Golay, Fast Fourier Transform, and soft threshold method. The evaluation results of denoising effect for the ME-C 4D simulation signal show that using Daubechies 5 (db5) wavelet at a decomposition level 4 can produce the best performance. Microseismic and streaming potential data from laboratory tests were used for comparison and showed similar trends as in the synthetic data analysis.A signal denoising method using improved wavelet threshold function is presented for microchip electrophoresis based on capacitively coupled contactless conductivity detection (ME-C 4D) device. Also, the EB method outperformed the soft and hard thresholding methods in general because the wavelet representation is not sparse at the coarsest levels, which leads to poor estimation of the noise variance by the thresholding methods. Quantitative comparison of the error between the true and estimated signal revealed that the wavelet-based methods outperformed the Wiener estimator in most cases. Four synthetic data sets, originally designed by Donoho and Johnstone to isolate and mimic various features found in real signals, were corrupted with correlated Gaussian noise to test the various denoising methods. Both real and synthetic data sets were used to compare the Wiener estimator in the Fourier domain, soft thresholding, hard thresholding, and the EB wavelet-based estimators. The Fourier-based technique used for comparison is the classical Wiener estimator, and the wavelet-based techniques tested include soft and hard wavelet thresholding and the empirical Bayes (EB) method.
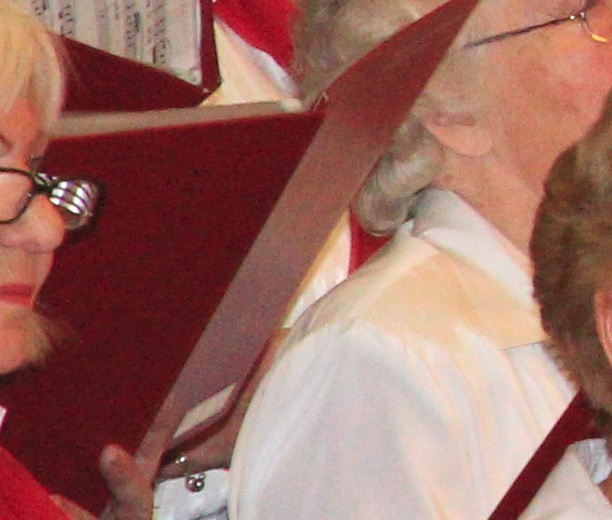
In this paper, we compare Fourier-based and wavelet-based denoising techniques applied to both synthetic and real experimental geophysical data.
